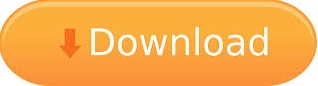
He lives by: “Why put off until later what can be completed in the present moment?”. Chris uses an unwavering sense of urgency while taking care of business. He seeks out the best and brightest in the industry and provides guidance, flexibility and availability to allow all to be the best that they can be and to grow professionally and as individuals. He also holds memberships in the Seminole County Chamber of Commerce, and the International Association of Electrical Inspectors.Ĭhris has strong ties to his family which he carries over into his work, treating those who work for him as part of the “K&K Family”. Chris’s brightest shining moment professionally was becoming a state certified Electrical Contractor and he (and K&K) is now licensed over a dozen states. In 2014 Chris became President of K&K Electric, growing the company to the current industry-leading electrical contracting company it is today. In 1998 he became Vice-President of K&K Electric, Inc. doi: 10.1377/ has held various leadership roles in the electrical industry over the past 26 years. Big data and new knowledge in medicine: the thinking, training, and tools needed for a learning health system. Big data in health care: using analytics to identify and manage high-risk and high-cost patients. Integrating predictive analytics into high-value care: the dawn of precision delivery. Beyond genes and molecules - a precision delivery initiative for precision medicine.
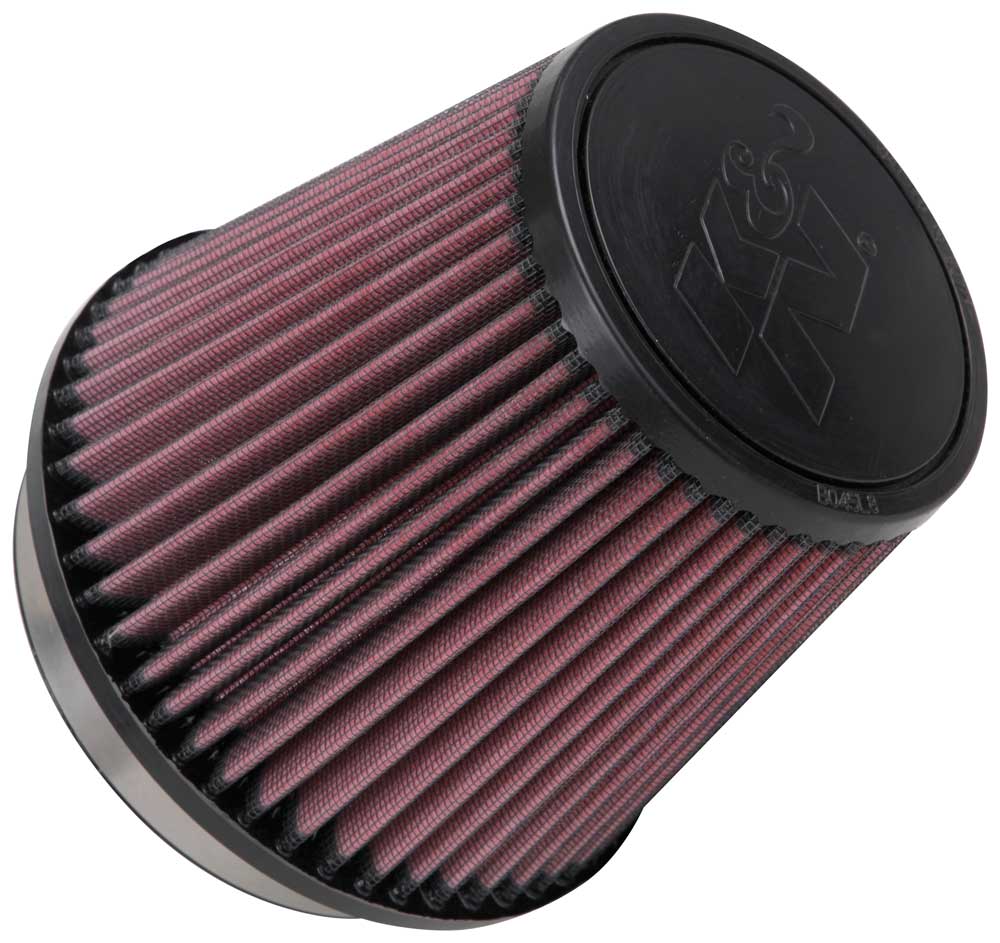
The Digital Universe: Driving Data Growth in Healthcare. In a case study of a particular prediction, we demonstrate that neural networks can be used to identify relevant information from the patient's chart. We believe that this approach can be used to create accurate and scalable predictions for a variety of clinical scenarios. These models outperformed traditional, clinically-used predictive models in all cases. Deep learning models achieved high accuracy for tasks such as predicting: in-hospital mortality (area under the receiver operator curve across sites 0.93-0.94), 30-day unplanned readmission (AUROC 0.75-0.76), prolonged length of stay (AUROC 0.85-0.86), and all of a patient's final discharge diagnoses (frequency-weighted AUROC 0.90). In the sequential format we propose, this volume of EHR data unrolled into a total of 46,864,534,945 data points, including clinical notes. We validated our approach using de-identified EHR data from two US academic medical centers with 216,221 adult patients hospitalized for at least 24 h.
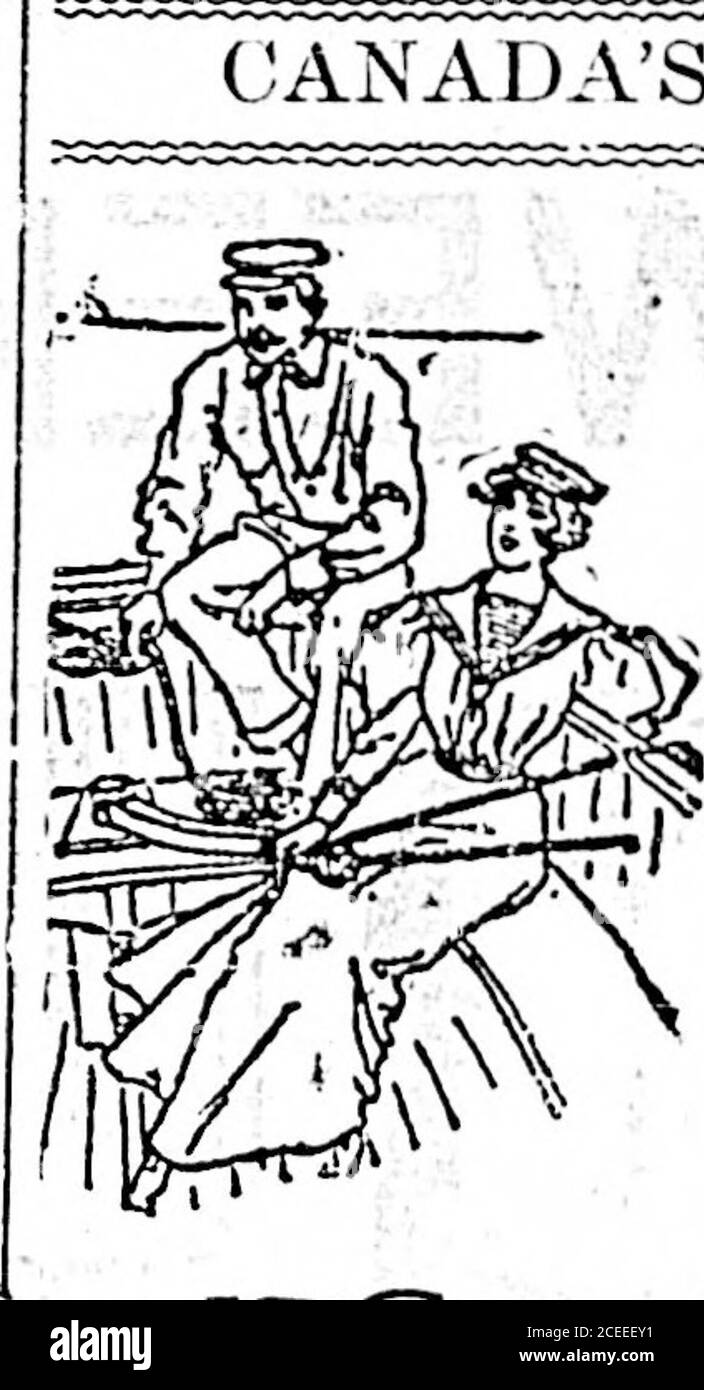
We demonstrate that deep learning methods using this representation are capable of accurately predicting multiple medical events from multiple centers without site-specific data harmonization. We propose a representation of patients' entire raw EHR records based on the Fast Healthcare Interoperability Resources (FHIR) format.

Constructing predictive statistical models typically requires extraction of curated predictor variables from normalized EHR data, a labor-intensive process that discards the vast majority of information in each patient's record. Predictive modeling with electronic health record (EHR) data is anticipated to drive personalized medicine and improve healthcare quality.
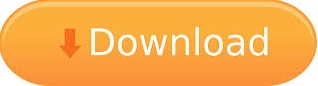